Machine Learning (ML): An enabler in a changing AML/CFT landscape
Christina Orfanidou 11:15 - 02 March 2023
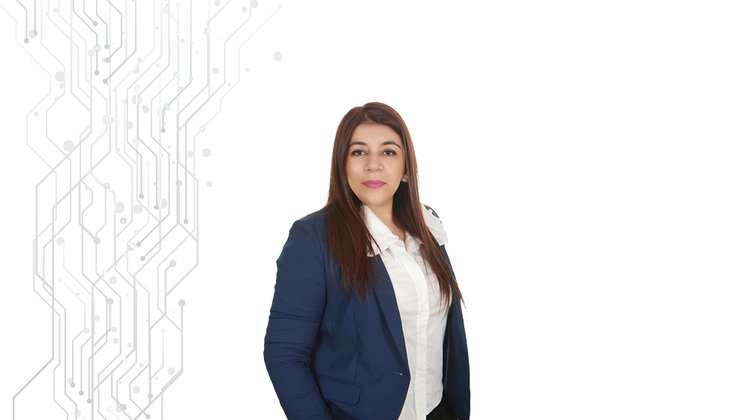
By Christina Orfanidou, Director, Risk Advisory, Deloitte
In order to stay up to date with the regulatory changes that the financial sector is facing, and which are increasing at a staggering rate, financial institutions are now turning to new technology solutions. Today, companies are embracing the use of advanced and predictive analytics, robotic process automation (RPA), artificial intelligence (AI), and cognitive technologies in their quest to improve business operations. The opportunity to harness new Regulatory Technology (RegTech) capabilities is now universally acknowledged by compliance professionals: by using RegTech to automate routine processes – thereby potentially improving quality and reducing costs – they can better respond to changing regulatory demands and apply analytics to advise business functions and determine areas of heightened regulatory risks. Over the past decade, regulators have asked financial institutions to modernise their businesses and many organisations have struggled with regulatory-driven transformations. RegTech established a solid foundation to overcome this and come up with solutions that are targeted at new and complex regulations combined with an overall reduction in cost compliance.
The EBA’s Analysis of RegTech in the EU Financial Sector highlights the potential of new technologies to benefit financial institutions, primarily pointing out the importance of enhanced risk management, advanced monitoring and sampling capabilities, and reduced human error as their key benefits. Additionally, according to the same report, FIs are keen to leverage RegTech solutions to enhance systems and data integration and to facilitate predictive analytics, enabling experts to focus on higher value-added tasks and processes. However, this may not always be easy to achieve in practice, as interoperability and integration are still reported as some of the main challenges faced by financial institutions in adopting RegTech solutions.
One of the areas receiving a great deal of attention in terms of technology applications is AML/CFT. As the volume of money laundering and other financial crimes increases worldwide, so too do the techniques used to evade their detection. While financial institutions are investing large sums of money every year to improve their defences against financial crime, the conventional rule-based approaches traditionally used for identifying and acting on suspicious or fraudulent activity do not yet match the sophistication of financial crime, making the fight against money laundering an ongoing challenge for compliance, monitoring and risk organisations. Now, however, there is an opportunity for financial institutions to get ahead. Recent advancements in machine learning (ML) are helping banks improve their AML programmes significantly, including the transaction monitoring element of these programmes. Incorporating fraud detection tools into customer identification and screening, adverse media screening and dynamic behavioural and transaction monitoring have been identified by EBA as the areas in which RegTech is most active in the AML/CFT market, from both a supply and demand perspective. These applications rely on a combination of device intelligence, behavioural analytics and a real-time multi-data point decision engine to detect potentially fraudulent artifacts and activities and provide decision support to compliance officers.
Defining a RegTech Strategy
Despite the emergence of these promising new technologies, operational challenges still need to be overcome if their true benefit is to be realised, including the challenge of roll-out across different countries, integration into legacy applications, integration challenges, as well as legal and regulatory obstacles.
As many of these challenges are institution-specific, the way each institution should approach and engage these emerging technologies needs to be tailored to its specific circumstances. Despite transformative advances in capability, technology still remains merely a tool, and finding the right tools for the job with a well-defined governance model for responsible use should be the overarching objective of any AML/CFT RegTech adoption initiative. AML/CFT RegTech adoption should be about defining a tailored approach for the unique characteristics of each institution. Amongst the important questions that companies should ask when preparing their AML/CFT RegTech strategy are the following:
- Does our institution have the right operating model to move to a more data-driven and forward-looking outlook with our compliance effort?
- Do we have the right people and skills to develop and lead a technology-driven approach to compliance or do we need external assistance?
- Should we develop the capabilities we need in-house or acquire externally?
- How do we stay ahead of the ways that regulators and peer companies are deploying advanced technologies and data analytics, so we don’t fall behind?
As RegTech technologies evolve and regulators become more knowledgeable in the value they can add to compliance activities, the possibility of automating processes and obtaining a view of organisations’ activities in real time could make RegTech an attractive option from a regulator’s perspective going forward. Financial institutions have to consider short-term vs. long-term approaches to their regulatory strategy and the adoption of new technologies needs to be carefully assessed and determined on the basis of cost and the ability to comply with incoming regulation. To achieve success in incorporating RegTech technologies into their AML/CFT activities, organisations need to ensure the chosen technologies are a good fit for their business activities and that the right skillset and mindset is in place to ensure adoption.
Deloitte’s AML/CFT Suite
Deloitte’s Custom AML/CFT Machine Learning Suite is a state-of-the-art, fast, and precise Machine Learning Fraud analytics system which enables the automatic identification of suspicious activity through various modules including:
- AML/CFT Model which aims to detect clients that should be flagged as suspicious. The model is trained to predict the probability that submitted information should be flagged, using information such as background information about the sender/receiver,their earlier behaviour and their transaction history.
- Transactional Model which is used to identify increasing risk soon since scores quickly reveal changing transactional patterns. Used for both underwriting and risk management on the existing portfolio.
CONTACT INFORMATION:
Christina Orfanidou
Director | Risk Advisory,
Tel: (+357) 25868838
e-mail: corfanidou@deloitte.com
(This interview first appeared in the February 2023 issue of GOLD magazine. Click here to view it.)